No matter which industry you’re in, safeguarding sensitive data and personally identifiable information (PII) is the core purpose of your security framework. Hush-Hush Data Masking Components use a variety of industrial-grade algorithms to meet or exceed all accepted standards for data privacy metrics like k-anonymity and l-diversity. But what does that mean exactly?
In this blog, we’ll tell you everything you need to know about this privacy model.
What Is K-Anonymity?
Simply put, k-anonymity is built on the idea that identifying an individual is more difficult when that individual’s sensitive data is hidden amongst a set of similar data. In other words, the information contained in the dataset could relate to anyone listed there.
For k-anonymity to be achieved, there needs to be at least k individuals in the dataset who share the same set of attributes. To put it another way, k refers to the number of times each combination of values appears in that data set. If k=3, then there are at least three sets of similar data contained in the database.
Methods for achieving k-anonymity include suppression and generalization. With suppression, certain data values are replaced by an asterisk '*'. With generalization, individual data values are replaced with a broader category.
K-anonymization can also be used to target indirect identifiers, that when combined, could potentially be used to identify an individual, such as age and gender. By using generalization, for example, the age value of 19 can be replaced with the generalized term ‘Under 20’, which decreases the risk of that value being used to identify someone directly.
What Is L-Diversity?
l-diversity is a benchmark measure to tell whether k-anonymization efforts are sufficient to avoid re-identification. l-diversity covers the weaknesses of k-anonymity, such as in a case where the number of k-individuals does not anonymize corresponding sensitive data that should be hidden. K-anonymity is achieved when the values of sensitive attributes are well-represented in a data set.
Hush-Hush Data Masking Components provide a high level of protection and allow you to de-identify both direct and identifiers, with both fixed and generic algorithms. Request your free trial today.
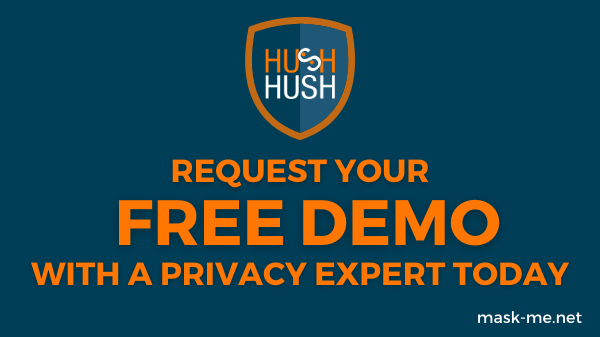